Accelerating GW calculations through machine-learned dielectric matrices
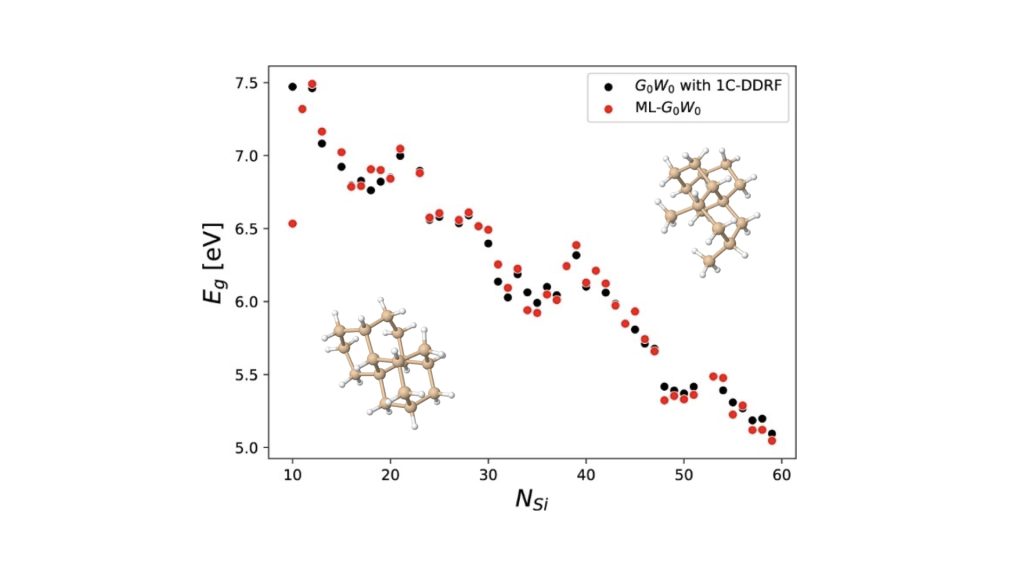
The interaction of nanoparticles with light plays an important role in many applications, for example solar cells or photocatalysis.
The fundamental quantity that determines if a nanoparticle can absorb light is the energy gap between its highly occupied orbital and its lowest unoccupied orbital. Predicting such energy gaps is notoriously difficult: widely used density-functional theory often significantly underestimates the energy gap (this is known as the band gap problem of DFT), while accurate many-body techniques, such as the GW approach, are numerically highly demanding and difficult to apply to large nanoparticles.
To overcome this problem, researchers at the TYC have developed a machine learning approach to accelerate GW calculations for nanoparticles. In this new method, we use a neural network to predict the dielectric matrix of the nanoparticle which determines how electrons interact with each other. This machine-learned dielectric matrix is then used input into the GW calculation. The method, which we called ML-GW, gives very good agreement with the full calculation, but at a fraction of the numerical cost.
Authors: Mario G. Zauchner, Andrew Horsfield, Johannes Lischner