
- This event has passed.
TYC AI/ML Interest Group Seminar: advances in machine learning for electrochemical systems
18 February 2025 @ 4:00 pm – 6:00 pm
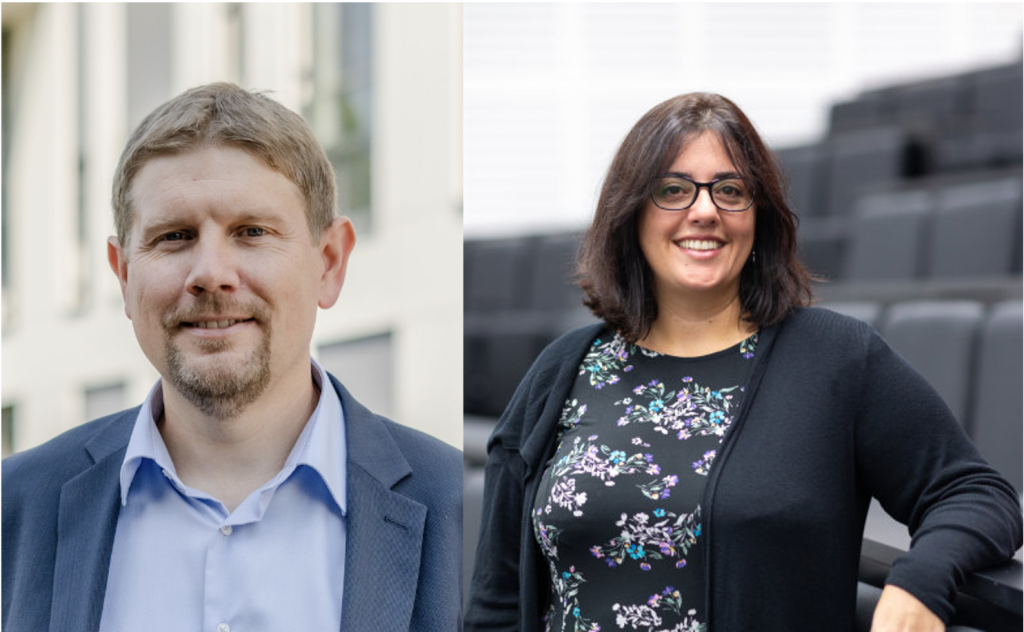
Jörg Behler, Ruhr University Bochum & Clotilde Cucinotta, Imperial College London
Atomistic Simulations with High-Dimensional Neural Network Potentials
Jörg Behler – Lehrstuhl für Theoretische Chemie II, Ruhr-Universität Bochum, Germany and Research Center Chemical Sciences and Sustainability, Research Alliance Ruhr, Germany
In recent years, there has been tremendous progress in the development of interatomic potentials employing machine learning. High-dimensional neural network potentials (HDNNP) are an important class of machine learning potentials, which allow to combine the accuracy of electronic structure calculations with the efficiency of simple empirical potentials enabling large-scale simulations. HDNNPs can be classified into four generations, which allow to study different types of systems and physical phenomena. In this talk, an overview about the methodical evolution of HDNNPs will be given along with typical example applications to condensed systems with a particular focus on chemical processes at interfaces.
Exploring the Pt(111)-Electrolyte Interface Under Applied Potentials with Ab Initio Molecular Dynamics
Clotilde Cucinotta, Imperial College London
In this talk, I will discuss some complexities in the simulation of electrified interfaces at the nanoscale, focusing on the impact of applied potentials on their physicochemical properties. My approach is based on the development of highly realistic ab initio molecular dynamics models of charged electrode-electrolyte interfaces under bias. I will discuss recent advancements in modelling the double layer of the electrified Pt(111)-electrolyte interface, particularly in terms of its response to the applied electrode potential. This is achieved through the application of electrode-charging and potential control methodologies developed in my group. If time permits, I will discuss how the how insights from molecular electronics can lead to a more sophisticated understanding of electrochemical phenomena.