
- This event has passed.
TYC Soiree: Bio Interest Group – Christian Jorgensen, University of Portsmouth & Matteo T. Degiacomi, University of Edinburgh
Venue: Roberts Building 421, UCL
12 December 2024 @ 3:00 pm – 5:00 pm
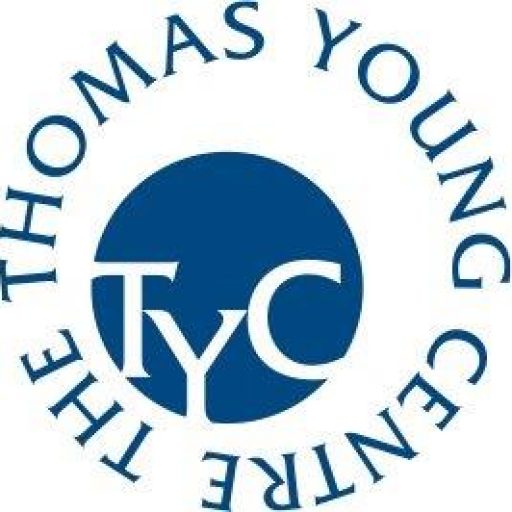
For anyone attending online:
https://ucl.zoom.us/j/91099194419?pwd=5QQfxercjapHZn3GCJltojEV90jcQ9.1
Meeting ID: 910 9919 4419
Passcode: 825356
Computational Models of Complex Membranes For Drug Delivery – Christian Jorgensen, University of Portsmouth
The use of computational models powered by Molecular Dynamics (MD) simulations has allowed for the construction of atomic-detail models of biophysical systems of interest. Here we demonstrate the use of MD simulations to construct a model of the blood-brain barrier endothelial cell membrane, and the human Stratum Corneum skin membrane. We show permeability simulations across these systems and elucidate the thermodynamics of transport for libraries of compounds.
Christian Jorgensen studied chemistry at Oxford University, followed by a PhD in Chemistry at King’s College London. He was a Postdoctoral Fellow with Peter Searson at Johns Hopkins, followed by a Postdoc at Georgetown University in Washington DC with Peter Olmsted. He was a Marie Sklodowska Curie Fellow at Aarhus University in Denmark, where he worked on multidrug resistance. He joined Portsmouth University as a Senior Lecturer in Pharmaceutical Sciences in 2024. His interest is in computational biophysics, with a focus on complex membranes and membrane proteins.
Learning (from) protein dynamics – Matteo T. Degiacomi, Durham University
Determining the different conformational states of a protein and the transition paths between them is key to fully understanding the relationship between biomolecular structure and function. I will discuss how a convolutional neural network can learn a continuous conformational space representation from example structures produced by molecular dynamics simulations. I will then show how such representation, obtained via our software molearn (1), can be leveraged to predict putative protein transition states (2), or to generate conformations useful in the context of flexible protein-protein docking (3).
1. S. C. Musson and M.T. Degiacomi, Molearn: a Python package streamlining the design of generative models of biomolecular dynamics. Journal of Open Source Software (2023)
2. V.K. Ramaswamy et al., Learning Protein Conformational Space with Convolutions and Latent Interpolations. Physical Review X (2021).
3. M.T. Degiacomi, Coupling Molecular Dynamics and Deep Learning to Mine Protein Conformational Space. Structure (2019).
Matteo Degiacomi obtained an MSc in Computer Science and a PhD in computational biophysics in Ecole Polytechnique Fédérale de Lausanne (EPFL). In 2013, funded by a Swiss National Science Foundation Early Postdoc Mobility Fellowship, he joined the research groups of Prof Justin Benesch and Prof Dame Carol Robinson FRS in the University of Oxford. In 2017 he obtained an EPSRC Junior Research Fellowship, allowing him to establish his independent research in Durham University, and in 2020 he was promoted to Associate Professor. In 2024 he moved to the University of Edinburgh, to take the position of Reader in Biomedical Artificial Intelligence joint between the Schools of Informatics and Chemistry.
Organised by:
Edina Rosta
e.rosta@ucl.ac.uk