Framework for efficient ab initio electronic structure with Gaussian Process States
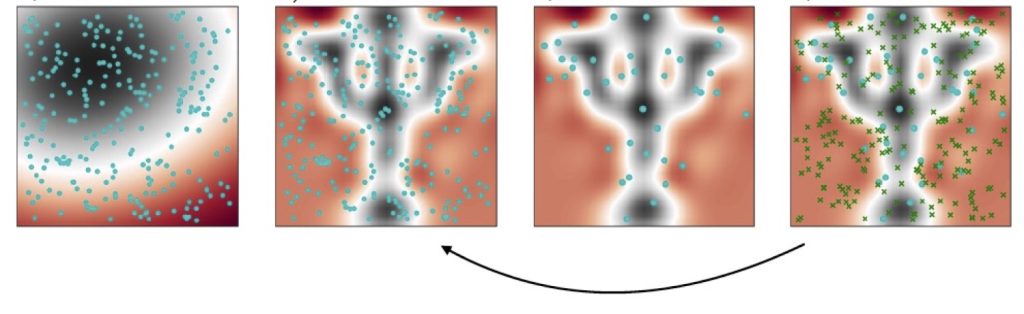
Machine learning and artificial intelligence is having a huge impact, but at their core they represent a set of models which can very accurately approximate high-dimensional functions. The wave function of a molecule or material describes the quantum mechanical probability amplitude of any given arrangement of electrons in the system. As such, it is an (exponentially) high-dimensional object, but its knowledge can enable us to calculate all properties of the system exactly, from its energetic barriers to structural properties and phase transitions. In this work, we apply the machine learning paradigm of Gaussian process regression to model the wave function of real interacting molecules and materials, achieving high accuracy. We manage to directly simulate a metal-to-insulator phase transition in a realistic model of a hydrogen material, driven solely by the strong quantum mechanical fluctuations in the system. This enables us to move beyond the parameterized phenomenological models that our understanding of condensed matter has relied on for so long, and move towards a first principles approach to material-specific quantum properties.
Authors: Yannic Rath and George H. Booth
https://journals.aps.org/prb/abstract/10.1103/PhysRevB.107.205119