Machine-learning structural reconstructions for accelerated point defect calculations
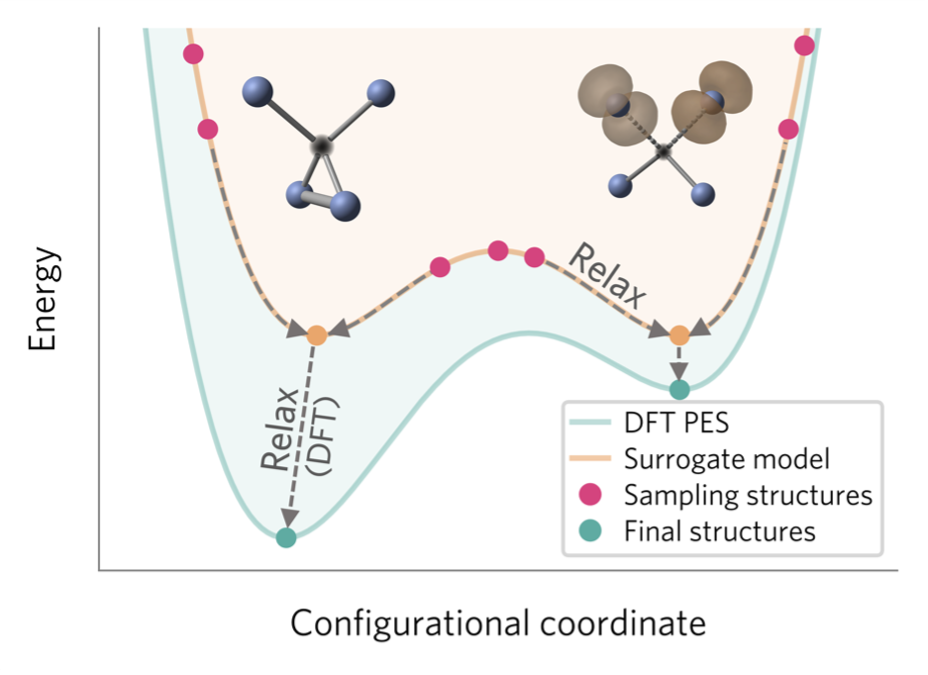
Accelerating defect modelling with Machine Learning.
Point defects make or break materials’ functionality. They limit the performance of solar cells, control charging and degradation rates in batteries, increase reaction rates in catalysts and can be used as qubits for quantum computers. Despite their impact on macroscopic properties, they are present in very dilute concentrations—which challenges experimental characterization, thus requiring a combination of experimental and theoretical methods to understand defect behaviour.
A key step in defect modelling involves finding the most stable defect geometry. However, global structure searching is computationally challenging for materials with complex defect landscapes (i.e. with many local minima, like alloys or disordered solids). Here, we tackle this limitation by harnessing a machine-learning surrogate model to qualitatively explore the defect structural landscape. By learning defect motifs in a family of related materials, the model successfully predicts favourable reconstructions for unseen defects in unseen crystals. Overall, our approach enables more accurate and faster defect studies for configurationally complex materials.
Authors: Irea Mosquera-Lois, Seán R. Kavanagh, Alex M. Ganose & Aron Walsh
npj Computational Materials; DOI: https://doi.org/10.1038/s41524-024-01303-9