SEM Image Processing Assisted by Deep Learning to Quantify Mesoporous γ-Alumina Spatial Heterogeneity and Its Predicted Impact on Mass TransferAn experimental flow regime map to dynamically structure gas-solid bubbling fluidized beds
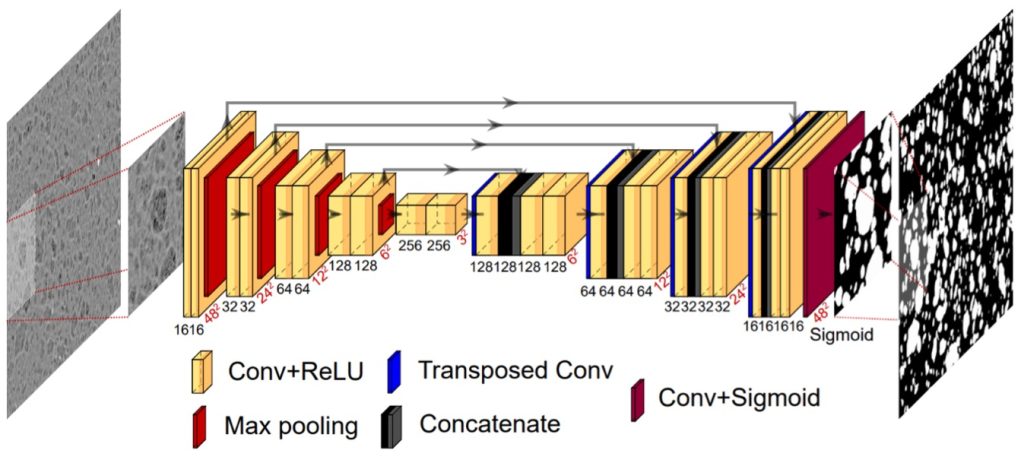
This article is part of Virtual Special Issue on Machine Learning in Physical Chemistry (Volume 2). It resulted from a collaborative PhD project by (now Dr) Aleksandra Glowska working with Professor Marc-Olivier Coppens at the UCL Centre for Nature-Inspired Engineering (CNIE), in collaboration with IFP Energies nouvelles (France).
The researchers investigated the spatial heterogeneity of γ-alumina, a widely used nanoporous catalyst support (catalytic reforming, steam reforming, hydrogenations, environmental catalysis…), and its impact on mass transfer. The study combines traditional characterisation methods with advanced scanning electron microscopy (SEM) imaging and deep learning semantic segmentation, to quantify variations in porosity within the material.
A key focus is on alumina inclusions, areas of differing porosity embedded within the alumina matrix. The researchers developed a novel methodology using machine learning to analyse SEM images and measure parameters relevant to mass transfer. They found that while spatial heterogeneity can influence the effective tortuosity factor (a measure of how easily molecules diffuse through the material), e.g., measured through pulsed-field gradient NMR, its impact was negligible for the specific aluminas studied, unless certain threshold levels of inclusion content and interphase porosity are met. The methods developed offer a general framework for characterising porous materials.
Authors: A. Glowska, E. Jolimaitre, A Hammoumi, M. Moreaud, L. Sorbier, C. de Faria Barros, V. Lefebvre and M-O. Coppens